1. Introduction to MINT-1T Dataset
Salesforce AI team has recently released MINT-1T, the largest open-source multimodal interleaved dataset to date. Key features of MINT-1T include:
- Scale: Contains 1 trillion tokens and 3.4 billion images, nearly 10 times larger than existing open-source datasets like OBELICS and MMC4.
- Diversity: Includes PDF documents and ArXiv papers in addition to HTML documents, significantly improving coverage of scientific documents.
- Quality: Employs a rigorous data processing pipeline, including language identification, content filtering, image processing, and deduplication.
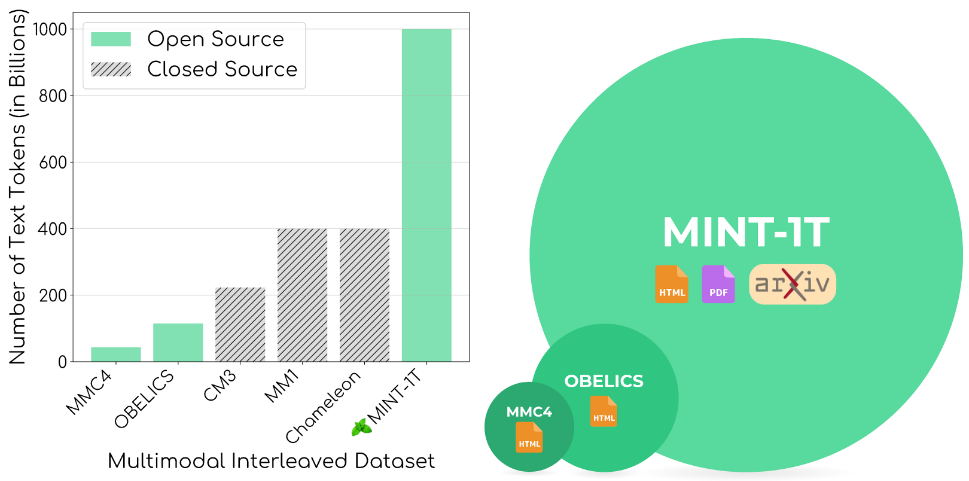
Figure 1: Comparison of MINT-1T dataset scale with other multimodal interleaved datasets
2. Dataset Construction Process
The construction of MINT-1T involved the following main steps:
2.1 Data Sources
- HTML documents: Extracted from CommonCrawl, processing time range from May 2017 to April 2024, using complete data from October 2018 to April 2024 and partial data from previous years.
- PDF documents: Extracted from CommonCrawl WAT files, time range from February 2023 to April 2024.
- ArXiv documents: Constructed interleaved documents using LaTeX source code.
2.2 Data Filtering and Processing
- Text quality filtering: Used FastText model for language identification, applied filtering rules from RefinedWeb and MassiveText.
- Image filtering: Removed images smaller than 150 pixels and larger than 20,000 pixels, applied NSFW detector.
- Deduplication: Used Bloom Filter for text deduplication, image deduplication based on SHA256 hash values.
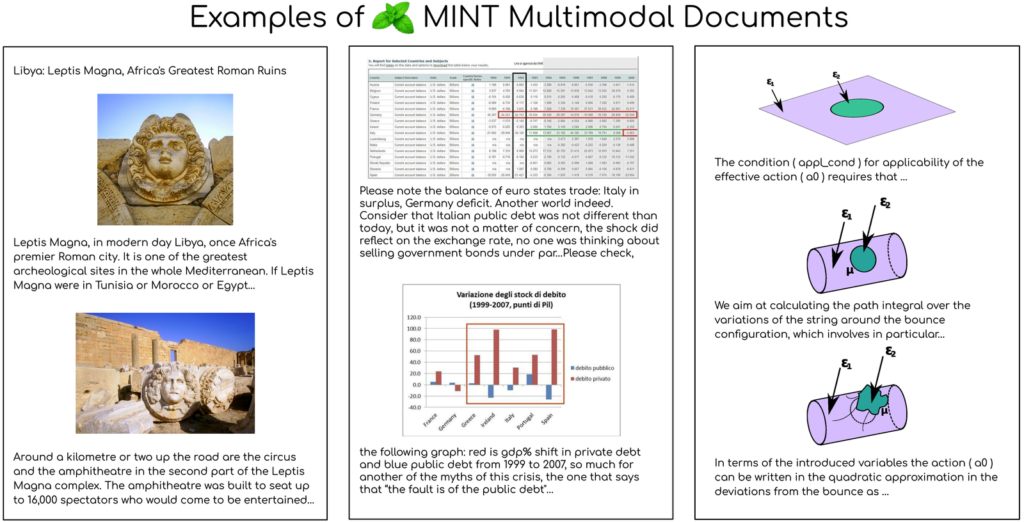
Figure 2: Examples of multimodal interleaved documents from HTML, PDF, and ArXiv in MINT-1T dataset
3. Model Experiment Results
The research team pretrained XGen-MM multimodal models using MINT-1T and conducted the following experiments:
- Pretraining data composition: 50% of tokens from HTML documents, the rest from PDF and ArXiv documents.
- Evaluation tasks: Image captioning and visual question answering benchmarks.
- Results: Models trained on MINT-1T outperformed those trained on the previous leading dataset OBELICS in visual question answering tasks.
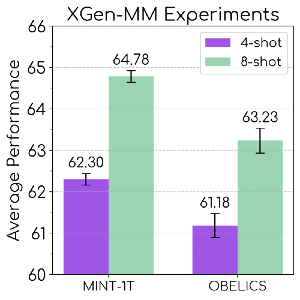
Figure 3: Performance comparison of XGen-MM models on MINT-1T and OBELICS datasets
4. Dataset Analysis
4.1 Document Composition Comparison
- Text token distribution: PDF and ArXiv documents in MINT-1T have significantly longer average lengths compared to HTML documents.
- Image density: PDF and ArXiv documents contain more images, with ArXiv samples having the highest image density.
4.2 Domain Coverage
- MINT-1T’s HTML subset shows broader domain coverage.
- The PDF subset is primarily focused on science and technology domains.
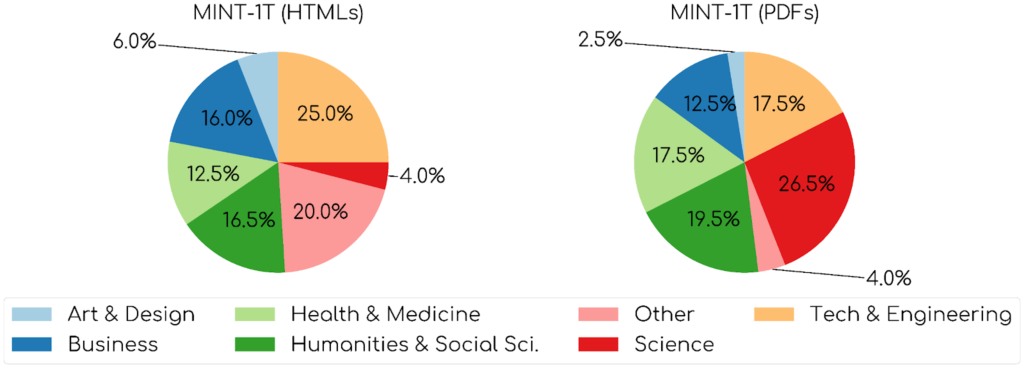
Figure 4: Domain distribution of HTML and PDF documents in MINT-1T dataset
4.3 In-context Learning Performance
- Models trained on MINT-1T outperform OBELICS baseline models across 1 to 8 examples.
4.4 Performance on Different Tasks
- Image captioning task: OBELICS dataset performs better.
- Visual question answering task: MINT-1T significantly outperforms other baselines.
- Multidisciplinary Multimodal Understanding and Reasoning (MMMU): MINT-1T excels in science and technology domains.
5. Future AI Development Trends and Insights
- The importance of large-scale multimodal data will continue to increase, potentially leading to similar datasets covering even broader domains and languages.
- Research in modality fusion will deepen, possibly exploring more complex interleaving methods including audio, video, and other modalities.
- AI models will better balance general capabilities with domain specialization.
- The balance between data quality and scale will receive more attention, with further development in data cleaning and quality control techniques.
- AI models’ contextual understanding and transfer learning capabilities are expected to further improve.
- More innovative model architectures optimized for multimodal tasks will emerge.
- Collaboration between open-source and commercial entities will promote rapid iteration and popularization of AI technologies.
- AI development will increasingly focus on balancing technological innovation with ethical responsibilities.
6. Conclusion
The release of MINT-1T marks a new stage in multimodal AI research, providing researchers with valuable resources and offering important references for the future direction of AI technology development. Based on this, we can expect multimodal AI to make greater breakthroughs in comprehension, creativity, and application scope.